일 | 월 | 화 | 수 | 목 | 금 | 토 |
---|---|---|---|---|---|---|
1 | 2 | |||||
3 | 4 | 5 | 6 | 7 | 8 | 9 |
10 | 11 | 12 | 13 | 14 | 15 | 16 |
17 | 18 | 19 | 20 | 21 | 22 | 23 |
24 | 25 | 26 | 27 | 28 | 29 | 30 |
31 |
Tags
- KONLPY
- 풀링층
- 완전연결층
- 텍스트 마이닝
- 시계열 분석
- 딥러닝
- 성능 최적화
- 카운트 벡터
- 프로그래머스
- 원-핫 인코딩
- 입력층
- NLTK
- 클러스터링
- 과적합
- 이미지 분류
- 생성모델
- 순환 신경망
- COLAB
- 합성곱 신경망
- 전이학습
- RNN
- 양방향 RNN
- 합성곱층
- 출력층
- 자연어 전처리
- 임베딩
- 망각 게이트
- cnn
- 코랩
- 코딩테스트
Archives
- Today
- Total
Colab으로 하루에 하나씩 딥러닝
텍스트 마이닝_ 1. BOW 기반의 텍스트 마이닝_1)카운트 기반/BOW의 문서 표현 본문
728x90
카운트 기반 문서 표현
- 문서의 의미를 반영해 벡터를 만드는 과정
- 단어의 빈도를 파악하여 문서의 내용을 파악 ex) 막대그래프, 워드 클라우드
- 전체 말뭉치에 한 번이라도 사용된 단어는 문서에 없더라도 특성에 포함하고 빈도를 0으로 주어 문서를 비교할 수 있도록 동일한 특성을 갖게 함
- 카운트 기반의 문서표현은 개별 문서가 아닌 말뭉치를 대상으로 함
- 문제점
- 문서를 표현하기 위해 너무나 많은 특성을 사용해야 함
- 대부분의 값이 0인 희소 벡터(sparse vector)를 사용하므로 저장공간과 연산 측면에 비효율적
BOW(Bags of Words)기반 문서 표현
- 단어별 카운트를 기반으로 문서로부터 특성을 추출하고 표현하는 방식
- 대상 문서를 BOW 기반으로 빈도를 계산하면 특성 벡터를 구할 수 있음
- 특성 벡터 추출 과정
- 문서 집합(말뭉치)를 토큰화, 정규화, 품사 태깅 등의 방법으로 의미가 있는 최소 단위의 리스트로 변환
- 특성 집합(특성 추출 대상이 되는 단어 집합)을 구성함. 구성 기준은 본인의 파라미터를 통해 단어들을 선별할 수 있음
- 각 문서별로 특성 추출 대상 단어들에 대해 단어의 빈도를 계산해 특성 벡터를 추출
- NLTK가 제공하는 영화 리뷰를 기반으로 BOW의 실습 진행
### 라이브러리 호출 및 영화 리뷰 문서의 id와 본문을 가져오고 문성의 내용과 토큰화
import nltk
nltk.download('punkt')
nltk.download('movie_reviews')
from nltk.corpus import movie_reviews
print('review count: ', len(movie_reviews.fileids())) # 영화 리뷰 문서의 id를 반환
print('*' *50)
print('samples of file ids: ', movie_reviews.fileids()[:10]) # id를 10개까지만 출력
print('*' *50)
fileid = movie_reviews.fileids()[0] # 첫번째 문서의 id를 반환
print('id of the firsr review : ', fileid)
print('*' *50)
# 첫번째 문서의 내용을 200자까지만 출력
print('first review content:\n', movie_reviews.raw(fileid)[:200])
print('*' *50)
# 첫번째 문서를 sentence tokenize한 결과 중 앞 두 문장
print('sentence tokenization result: ', movie_reviews.sents(fileid)[:2])
print('*' *50)
# 첫번째 문서를 word tokenize한 결과 중 앞 20개 단어
print('word tokenization result: ', movie_reviews.words(fileid)[:20])
print('*' *50)
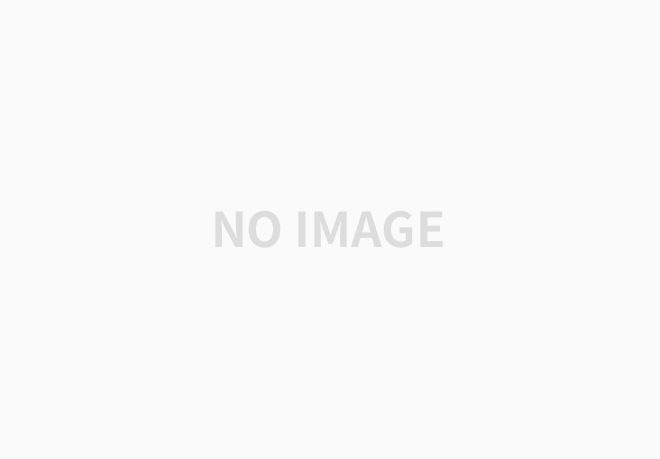
### 모든 문서의 id를 가져오고 words()로 토큰화
documents = [list(movie_reviews.words(fileid)) for fileid in movie_reviews.fileids()]
print(documents[0][:50]) # 첫째 문서의 앞 50개 단어를 출력
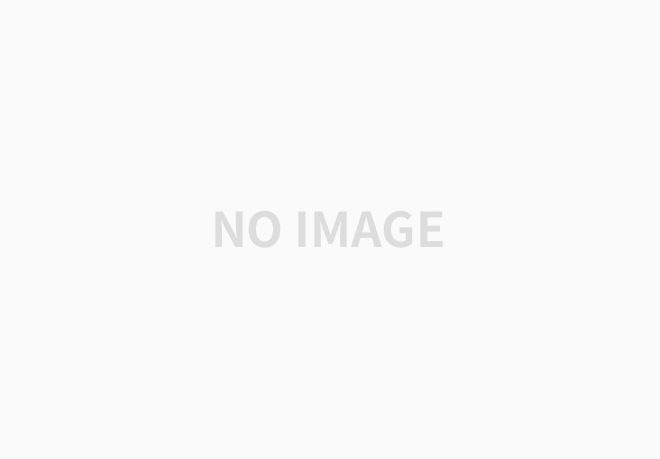
### 특성 집합을 만들기 위해 말뭉치 전체에서의 빈도를 계산하고 빈도가 높은 단어 확인
word_count = {}
for text in documents:
for word in text:
word_count[word] = word_count.get(word, 0) + 1
sorted_features = sorted(word_count, key=word_count.get, reverse=True)
for word in sorted_features[:10]:
print(f"count of '{word}': {word_count[word]}", end=', ')

### 토큰화에 대한 다시 전처리 실행(정규표현식 사용, NLTK 불용어 사전 사용)
import nltk
nltk.download('stopwords')
from nltk.tokenize import RegexpTokenizer
from nltk.corpus import stopwords # 일반적으로 분석 대상이 아닌 단어들
tokenizer = RegexpTokenizer("[\w']{3,}") # 정규표현식으로 토크나이저를 정의
english_stops = set(stopwords.words('english')) # 영어 불용어를 가져옴
# words() 대신 raw()로 원문을 가져옴
documents = [movie_reviews.raw(fileid) for fileid in movie_reviews.fileids()]
# stopwords의 적용과 토큰화를 동시에 수행
tokens = [[token for token in tokenizer.tokenize(doc) if token not in english_stops] for doc in documents]
word_count = {}
for text in tokens:
for word in text:
word_count[word] = word_count.get(word, 0) + 1
sorted_features = sorted(word_count, key=word_count.get, reverse=True)
print('num of features:', len(sorted_features))
for word in sorted_features[:10]:
print(f"count of '{word}': {word_count[word]}", end=', ')

### 빈도가 높은 상위 1000개의 단어만 추출해 features를 구성
word_features = sorted_features[:1000]
### word_features의 모든 단어에 대해 빈도를 계산해 리스트로 반환하는 함수 만들기(테스트)
# 주어진 document를 feature로 변환하는 함수, word_Features를 사용
def document_features(document, word_features):
word_count = {}
for word in document: # document에 있는 단어들에 대해 빈도수를 먼저 계산
word_count[word] = word_count.get(word, 0) + 1
features = []
# word_features의 단어에 대해 계산된 빈도수를 features에 추가
for word in word_features:
features.append(word_count.get(word, 0)) # 빈도가 없는 단어는 0을 입력
return features
word_features_ex = ['one', 'two','teen','couples','solo']
doc_ex = ['two', 'two', 'couples']
print(document_features(doc_ex, word_features_ex))

### 전체 리뷰 집합에 적용, 특성 집합 확인
feature_sets = [document_features(d, word_features) for d in tokens]
# 첫째 feature set의 내용을 앞 20개만 word_features의 단어와 함께 출력
for i in range(20):
print(f'({word_features[i]}, {feature_sets[0][i]})', end=', ')

참고: 박상언, 『파이썬 텍스트 마이닝 완벽 가이드』, 위키북스(2022)
'딥러닝_개념' 카테고리의 다른 글
텍스트 마이닝_ 1. BOW 기반의 텍스트 마이닝_3)나이브 베이즈 (0) | 2022.12.23 |
---|---|
텍스트 마이닝_ 1. BOW 기반의 텍스트 마이닝_2)코사인 유사도 (0) | 2022.12.21 |
자연어 전처리_3.임베딩_5) 한국어 임베딩 (0) | 2022.12.16 |
자연어 전처리_3.임베딩_4) 횟수/예측 기반 임베딩 (1) | 2022.12.15 |
자연어 전처리_3.임베딩_3) 예측 기반 임베딩 (1) | 2022.12.14 |